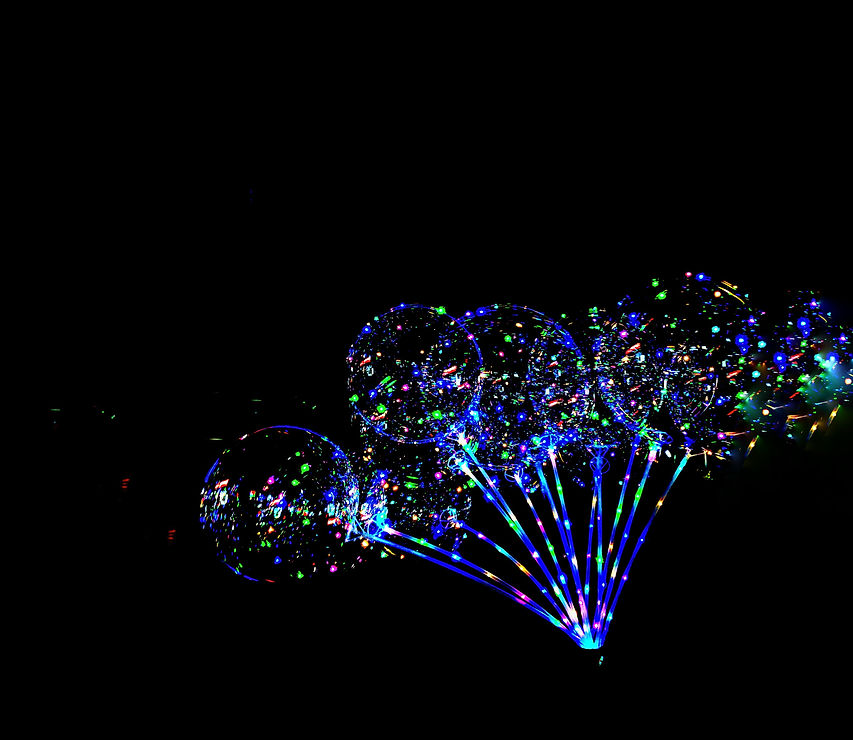
SAMIUL BASED SHUVO
Journey Towards The Creator
BIO
Personal Profile
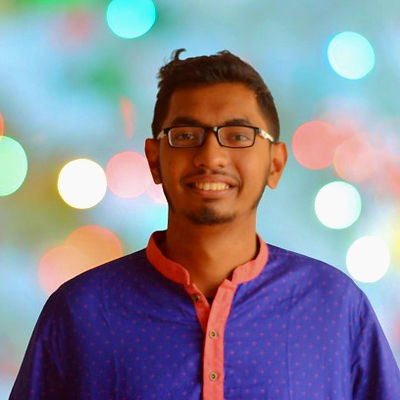
Throughout my life, I’ve approached every challenge with enthusiasm, creativity, and ceaseless desire to achieve success. This passion and drive have paved the way to countless opportunities, unique experiences and exceptional relationships both personally and professionally. If you’re interested in learning more about me, keep reading or get in touch.
EDUCATION
Learning and Living
January 2017Â - May 2021(expected)
BSC IN BIOMEDICAL ENGINEERING
BANGLADESH UNIVERSITY OF ENGINEERING AND TECHNOLOGY
Bangladesh University of Engineering and Technology , commonly known as BUET, is a public university in Bangladesh, which focuses on the study of engineering and architecture.Founded in 1912, it is the oldest institution for the study of engineering (initially it was a diploma school), architecture and urban planning in Bangladesh.
June 2014 - April 2016
BCIC COLLEGE
BCIC College is an educational institution Dhaka, Bangladesh.It is an institution operated by Bangladesh Chemical Industries Corporation. This institution is managed by the intelligent officer of Bangladesh Army.
January 2004 - March 2014
MONIPUR SCHOOL AND COLLEGE
Monipur High School & College is a secondary school and college located in Mirpur, Dhaka, Bangladesh. Around 35,500 students, including boys and girls, are currently studying at Monipur High School & College.
AREA OF INTEREST
Tricks of the Trade
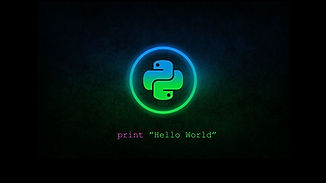
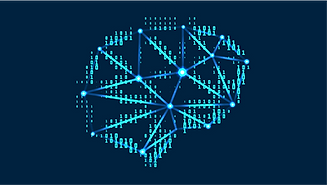
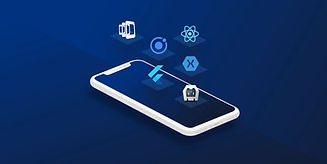
PROGRAMMING
Programming is the process of creating a set of instructions that tell a computer how to perform a task. From my high school, I was really fascinated about programming. The main reason is my love for logical thinking. It helps me to bind my imaginations and ideas with mathematical algorithms and simulate them. I am quite proficient at C, C++, Python, MATLAB.
DATA SCIENCE AND MACHINE LEARNING
Data science is an umbrella term that encompasses data analytics, data mining, machine learning, and several other related disciplines.Though i am new in this field ,but the capability of its really amused me.I am learning everyday newer and newer things and how much flexible it is!I am currently working with keras and Pytorch in my projects.I am also proficient in some data analysis and visualization tools like seaborn ,pandas,matplotlib.
APP DEVELOPMENT
App Development refers to the creation of computer applications for use on mobile devices such as tablets, smartphones and smart watches.I am taking App Development as an important tool for making real world and user end implementation of my research works.I am still not very comfortable in this field with the knowledge of Flutter and java.Currently i am learning api and backend data structure with SQL.
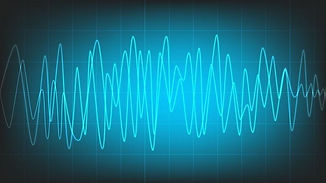
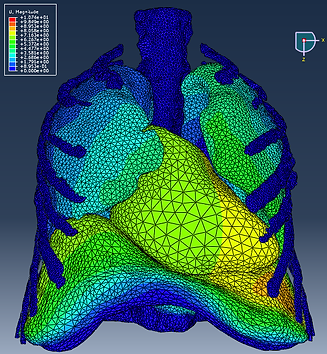
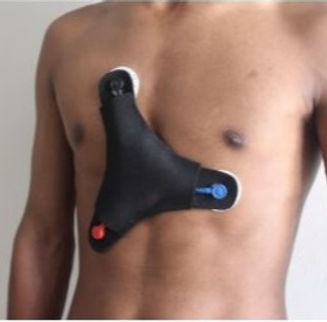
SIGNAL PROCESSING
Signal processing is an electrical engineering subfield that focuses on analysing, modifying, and synthesizing signals such as sound, images, and scientific measurements.In my recent works several signal processing techniques were involved i.e. cwt,emd.
SIMULATION ENGINEERING
A simulation is an approximate imitation of the operation of a process or system; that represents its operation over time.Several physiological systems and their aspects are needed to simulation.I am quite comfortable with Solidworks , ANSYS ,Simulink and use them in my several projects.
INSTRUMENTATION AND SENSOR
Instrumentation is a collective term for measuring instruments that are used for indicating, measuring and recording physical quantities.I am really love to play with different sensors and do different real world projects.I am currently working with ECG,PPG,EMG sensors and familiar with 3d printing. I worked in several projects as well i.e. wireless ECG acquisition devices,ventilator development etc.
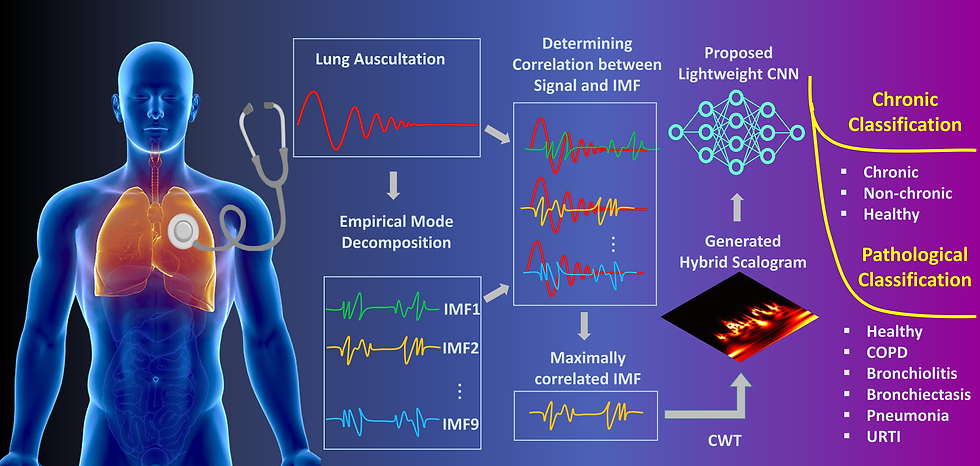
RESEARCH WORK
What I’ve Done

A LOW-COST, LOW-ENERGY WEARABLE ECG SYSTEM WITH CLOUD-BASED ARRHYTHMIA DETECTION
Continuously monitoring the Electrocardiogram (ECG) is an essential tool for Cardiovascular Disease (CVD) patients. In low-resource countries, the hospitals and health centers do not have adequate ECG systems, and this unavailability exacerbates the patients’ health condition. Lack of skilled physicians, limited availability of continuous ECG monitoring devices, and their high prices, all lead to a higher CVD burden in the developing countries. To address these challenges, we present a low-cost, low-power, and wireless ECG monitoring system with deep learning-based automatic arrhythmia detection. Flexible fabric-based design and the wearable nature of the device enhances the patient’s comfort while facilitating continuous monitoring. An AD8232 chip is used for the ECG Analog FrontEnd (AFE) with two 450 mi-Ah Li-ion batteries for powering the device. The acquired ECG signal can be transmitted to a smartdevice over Bluetooth and subsequently sent to a cloud server for analysis. A 1-D Convolutional Neural Network (CNN) based deep learning model is developed that provides an accuracy of 94.03% in classifying abnormal cardiac rhythm on the MIT-BIH Arrhythmia Database.
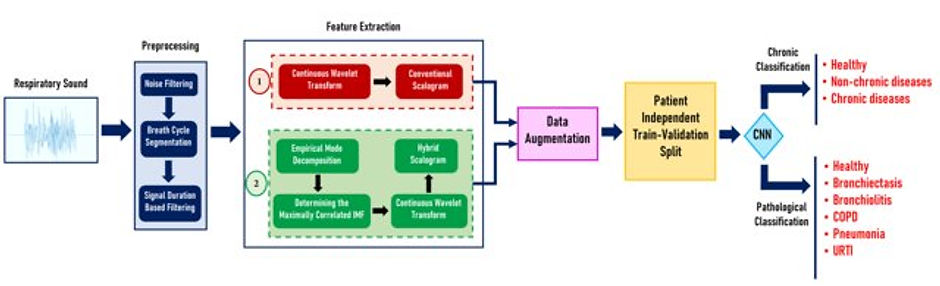
A LIGHTWEIGHT CNN MODEL FOR DETECTING RESPIRATORY DISEASES FROM LUNG AUSCULTATION SOUNDS USING EMD-CWT-BASED HYBRID SCALOGRAM
Listening to lung sounds through auscultation is vital in examining the respiratory system for abnormalities. Automated analysis of lung auscultation sounds can be beneficial to the health systems in low-resource settings where there is a lack of skilled physicians. In this work, we propose a lightweight convolutional neural network (CNN) architecture to classify respiratory diseases using hybrid scalogram-based features of lung sounds. The hybrid scalogram features utilize the empirical mode decomposition (EMD) and continuous wavelet transform (CWT). The proposed scheme's performance is studied using a patient independent train-validation set from the publicly available ICBHI 2017 lung sound dataset. Employing the proposed framework, weighted accuracy scores of 99.20% for ternary chronic classification and 99.05% for six-class pathological classification are achieved, which outperform well-known and much larger VGG16 in terms of accuracy by 0.52% and 1.77% respectively. The proposed CNN model also outperforms other contemporary lightweight models while being computationally comparable.
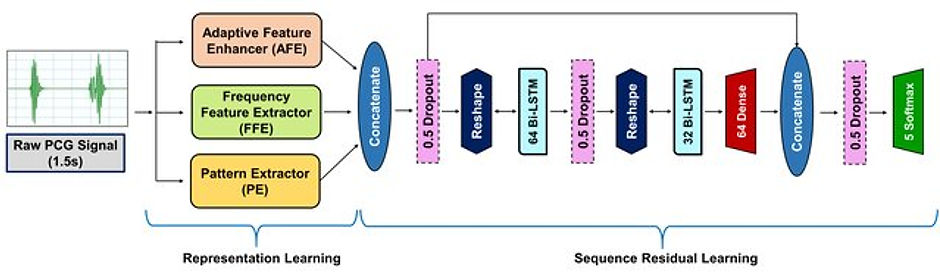
CARDIOXNET: A NOVEL LIGHTWEIGHT CRNN FRAMEWORK FOR CLASSIFYING CARDIOVASCULAR DISEASES FROM PHONOCARDIOGRAM RECORDINGS
The alarmingly high mortality rate and increasing global prevalence of cardiovascular diseases (CVDs) signify the crucial need for early detection schemes. Phonocardiogram(PCG) signals has been historically applied in this domain owing to its simplicity and cost-effectiveness. However, insufficiency of expert physicians and human subjectivity affect the applicability of this technique, especially in the low-resource settings. For resolving this issue, in this paper, we introduce CardioXNet,a novel lightweight CRNN architecture for automatic detection of five classes of cardiac auscultation namely normal, aortic stenosis, mitral stenosis, mitral regurgitation and mitral valve prolapse using raw PCG signal. The process has been automated by the involvement of two learning phases namely, representation learning and sequence residual learning. The first phase mainly focuses on automated feature extraction and it has been implemented in a modular way with three parallel CNN pathways i.e., frequency feature extractor (FFE), pattern extractor (PE) and adaptive feature extractor (AFE). 1D-CNN based FFE and PE respectively learn the coarse and fine-grained features from the PCG while AFE explores the salient features from variable receptive fields involving 2D-CNN based squeezeexpansion. Thus, in the representation learning phase, the network extracts efficient time-invariant features and converges with great rapidity. In the sequential residual learning phase,because of the bidirectional-LSTMs and the skip connection, the network can proficiently extract temporal features. The obtained results demonstrate that the proposed end-to-end architecture yields outstanding performance in all the evaluation metrics compared to the previous state-of-the-art methods with up to 99.6% accuracy, 99.6% precision, 99.6% recall and 99.4% F1-score on an average while being computationally comparable.
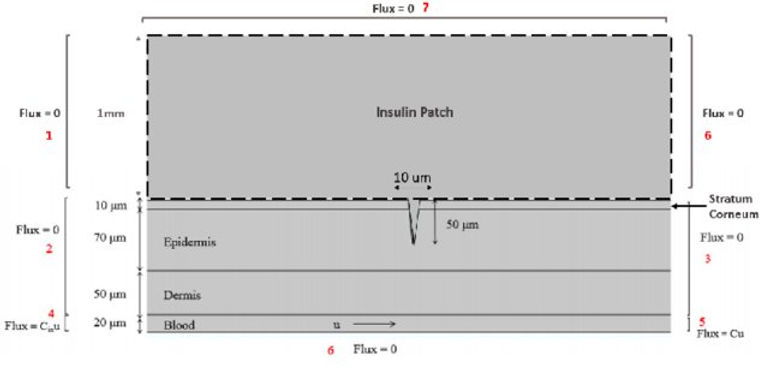
THE EFFECTS OF ANATOMICAL POSITIONS ON TRANSDERMAL INSULIN DELIVERY MODEL USING MICRONEEDLE PATCH
Transdermal delivery is a recent phenomenon in terms of smart drug delivery. Diabetes has been one of the leading causes of death throughout the world. Besides death, diabetic patients need to take regular doses of insulin for maintaining normoglycemic conditions. Transdermal patches can be limited in their efficacy for delivering insulin as it has a high molecular weight. Personalization of insulin delivery patches can result in optimal drug delivery, varying anatomical position, and controlling insulin uptake within the body. The objective of this work was to quantify the change in insulin concentration while using a transdermal insulin patch with multiple microneedles along with convectional blood flow. To this end, COMSOL has been used for insulin concentration simulation at multiple locations of the body and at different skin positions. It has been found, shoulder and forearm are the suitable locations for bolus insulin delivery while the abdomen is better suited for basal insulin delivery. The simulation showed a 66% difference in insulin concentration at blood flow between shoulder and abdomen location. This work can improve our understanding of insulin patch placement and thus transdermal therapy. Here, the validated model can work as a great starting block for further simulation including glucose control simulations.
… Read more

A ROBUST DEEP LEARNING FRAMEWORK FOR REAL-TIME DENOISING OF HEART SOUND
The heart sound signal captured via a digital stethoscope is often corrupted with environmental and physiological noise, altering its salient and critical properties. The problem is exacerbated in crowded low-resource hospital settings with high noise levels. In this study, inspired by the heart sound’s quasi-periodic nature, we present a novel deep encoder-decoderbased LU-Net architecture to suppress ambient hospital and internal lung sound noises that corrupt the heart sounds. Training is done using a large benchmark PCG dataset mixed with physiological noise, i.e., breathing sounds. Two different noisy datasets were created for experimental evaluation by mixing unseen lung sounds and hospital ambiance noises with the clean heart sound recordings. We also use the inherently noisy portion of the PASCAL heart sound dataset for evaluation. Experimental results show that the proposed framework can effectively suppress background noise in both real-world and synthetically generated noisy heart sound recordings, improving the signal-to-noise ratio (SNR) level by 5.575 dB on an average using only 1.32 M parameters. The study reveals that the proposed model outperforms the current state-of-the-art U-Net model with an average SNR improvement of 5.613 dB and 5.537 dB in the presence of lung sound and hospital noise, respectively. LU-Net also outperformed the state-of-the-art Fully Convolutional Network (FCN) by 1.750 dB and 1.748 dB for lung sound and hospital noise conditions, respectively. In addition, the proposed denoising method model improves classification accuracy by 38.93% in the noisy portion of the PASCAL heart sound dataset. The proposed enhancement scheme can thus play a vital role in deploying automated cardiac screening systems in low-resource and underserved communities.
